6. YOLOv8 追踪计数#
YOLOv8 是 Ultralytics 开发的 YOLO(You Only Look Once)目标检测和图像分割模型的最新版本。YOLOv8 模型设计为快速、准确且易于使用,使其成为广泛目标检测和图像分割任务的绝佳选择。它可以在大型数据集上进行训练,并且能够在从 CPU 到 GPU 的各种硬件平台上运行。
在本教程中,我们将介绍如何使用 YOLOv8 进行目标检测和图像分割,并展示如何使用 ByteTrack 进行目标追踪和计数。我们将使用 Roboflow 提供的预训练模型和数据集,以便您可以立即开始使用。
专业建议:使用 GPU 加速#
如果您在 Google Colab 中运行此笔记本,请导航到 编辑
-> 笔记本设置
-> 硬件加速器
,将其设置为 GPU
,然后单击 保存
。这将确保您的笔记本使用 GPU,从而显著加快模型训练速度。
本教程的步骤#
在本教程中,我们将涵盖以下内容:
开始之前
下载视频
安装 YOLOv8
安装 ByteTrack
安装 Roboflow Supervision
跟踪工具
加载预训练的 YOLOv8 模型
预测并标注单帧图像
预测并标注整个视频
让我们开始吧!
开始之前#
让我们确保我们可以访问 GPU。我们可以使用 nvidia-smi
命令来检查。如果遇到任何问题,请导航到 编辑
-> 笔记本设置
-> 硬件加速器
,将其设置为 GPU
,然后单击 保存
。
!nvidia-smi
'nvidia-smi' 不是内部或外部命令,也不是可运行的程序
或批处理文件。
import os
HOME = os.getcwd()
print(HOME)
D:\3000-code\deeplearning\DeepLearning2023\Deeplearning\chapters\chpt2
下载视频#
%cd {HOME}
!wget --load-cookies /tmp/cookies.txt "https://docs.google.com/uc?export=download&confirm=$(wget --quiet --save-cookies /tmp/cookies.txt --keep-session-cookies --no-check-certificate 'https://docs.google.com/uc?export=download&id=1pz68D1Gsx80MoPg-_q-IbEdESEmyVLm-' -O- | sed -rn 's/.*confirm=([0-9A-Za-z_]+).*/\1\n/p')&id=1pz68D1Gsx80MoPg-_q-IbEdESEmyVLm-" -O vehicle-counting.mp4 && rm -rf /tmp/cookies.txt
D:\3000-code\deeplearning\DeepLearning2023\Deeplearning\chapters\chpt2
D:\Program Files\Python39\lib\site-packages\IPython\core\magics\osm.py:417: UserWarning: using dhist requires you to install the `pickleshare` library.
self.shell.db['dhist'] = compress_dhist(dhist)[-100:]
'wget' 不是内部或外部命令,也不是可运行的程序
或批处理文件。
SOURCE_VIDEO_PATH = f"{HOME}/vehicle-counting.mp4"
安装 YOLOv8#
⚠️ YOLOv8 仍在积极开发中。几乎每周都会引入重大更改。我们努力使我们的 YOLOv8 笔记本与库的最新版本兼容。最后一次测试于 2023年1月23日 进行,使用的版本为 YOLOv8.0.17。
# Pip install method (recommended)
!pip install "ultralytics<=8.3.40"
from IPython import display
display.clear_output()
import ultralytics
ultralytics.checks()
---------------------------------------------------------------------------
ModuleNotFoundError Traceback (most recent call last)
Cell In[5], line 8
5 from IPython import display
6 display.clear_output()
----> 8 import ultralytics
9 ultralytics.checks()
ModuleNotFoundError: No module named 'ultralytics'
安装 ByteTrack#
ByteTrack 是一个优秀的追踪器,但它的打包方式有些问题。我们需要克服一些困难才能使其与 YOLOv8 协同工作。
%cd {HOME}
!git clone https://github.com/ifzhang/ByteTrack.git
%cd {HOME}/ByteTrack
# workaround related to https://github.com/roboflow/notebooks/issues/80
!sed -i 's/onnx==1.8.1/onnx==1.9.0/g' requirements.txt
!pip3 install -q -r requirements.txt
!python3 setup.py -q develop
!pip install -q cython_bbox
!pip install -q onemetric
# workaround related to https://github.com/roboflow/notebooks/issues/112 and https://github.com/roboflow/notebooks/issues/106
!pip install -q loguru lap thop
from IPython import display
display.clear_output()
import sys
sys.path.append(f"{HOME}/ByteTrack")
import yolox
print("yolox.__version__:", yolox.__version__)
yolox.__version__: 0.1.0
from yolox.tracker.byte_tracker import BYTETracker, STrack
from onemetric.cv.utils.iou import box_iou_batch
from dataclasses import dataclass
@dataclass(frozen=True)
class BYTETrackerArgs:
track_thresh: float = 0.25
track_buffer: int = 30
match_thresh: float = 0.8
aspect_ratio_thresh: float = 3.0
min_box_area: float = 1.0
mot20: bool = False
安装 Roboflow Supervision#
!pip install supervision==0.1.0
from IPython import display
display.clear_output()
import supervision
print("supervision.__version__:", supervision.__version__)
supervision.__version__: 0.1.0
from supervision.draw.color import ColorPalette
from supervision.geometry.dataclasses import Point
from supervision.video.dataclasses import VideoInfo
from supervision.video.source import get_video_frames_generator
from supervision.video.sink import VideoSink
from supervision.notebook.utils import show_frame_in_notebook
from supervision.tools.detections import Detections, BoxAnnotator
from supervision.tools.line_counter import LineCounter, LineCounterAnnotator
跟踪工具#
不幸的是,我们必须手动将模型生成的边界框与追踪器创建的边界框进行匹配。
from typing import List
import numpy as np
# converts Detections into format that can be consumed by match_detections_with_tracks function
def detections2boxes(detections: Detections) -> np.ndarray:
return np.hstack((
detections.xyxy,
detections.confidence[:, np.newaxis]
))
# converts List[STrack] into format that can be consumed by match_detections_with_tracks function
def tracks2boxes(tracks: List[STrack]) -> np.ndarray:
return np.array([
track.tlbr
for track
in tracks
], dtype=float)
# matches our bounding boxes with predictions
def match_detections_with_tracks(
detections: Detections,
tracks: List[STrack]
) -> Detections:
if not np.any(detections.xyxy) or len(tracks) == 0:
return np.empty((0,))
tracks_boxes = tracks2boxes(tracks=tracks)
iou = box_iou_batch(tracks_boxes, detections.xyxy)
track2detection = np.argmax(iou, axis=1)
tracker_ids = [None] * len(detections)
for tracker_index, detection_index in enumerate(track2detection):
if iou[tracker_index, detection_index] != 0:
tracker_ids[detection_index] = tracks[tracker_index].track_id
return tracker_ids
加载 pre-trained YOLOv8 模型#
# settings
MODEL = "yolov8x.pt"
from ultralytics import YOLO
model = YOLO(MODEL)
model.fuse()
Downloading https://github.com/ultralytics/assets/releases/download/v0.0.0/yolov8x.pt to yolov8x.pt...
100%|██████████| 131M/131M [00:00<00:00, 234MB/s]
YOLOv8x summary (fused): 268 layers, 68200608 parameters, 0 gradients
预测单帧图像#
# dict maping class_id to class_name
CLASS_NAMES_DICT = model.model.names
# class_ids of interest - car, motorcycle, bus and truck
CLASS_ID = [2, 3, 5, 7]
# create frame generator
generator = get_video_frames_generator(SOURCE_VIDEO_PATH)
# create instance of BoxAnnotator
box_annotator = BoxAnnotator(color=ColorPalette(), thickness=4, text_thickness=4, text_scale=2)
# acquire first video frame
iterator = iter(generator)
frame = next(iterator)
# model prediction on single frame and conversion to supervision Detections
results = model(frame)
detections = Detections(
xyxy=results[0].boxes.xyxy.cpu().numpy(),
confidence=results[0].boxes.conf.cpu().numpy(),
class_id=results[0].boxes.cls.cpu().numpy().astype(int)
)
# format custom labels
labels = [
f"{CLASS_NAMES_DICT[class_id]} {confidence:0.2f}"
for _, confidence, class_id, tracker_id
in detections
]
# annotate and display frame
frame = box_annotator.annotate(frame=frame, detections=detections, labels=labels)
%matplotlib inline
show_frame_in_notebook(frame, (16, 16))
0: 384x640 3 cars, 1 truck, 66.5ms
Speed: 14.1ms preprocess, 66.5ms inference, 22.4ms postprocess per image at shape (1, 3, 640, 640)
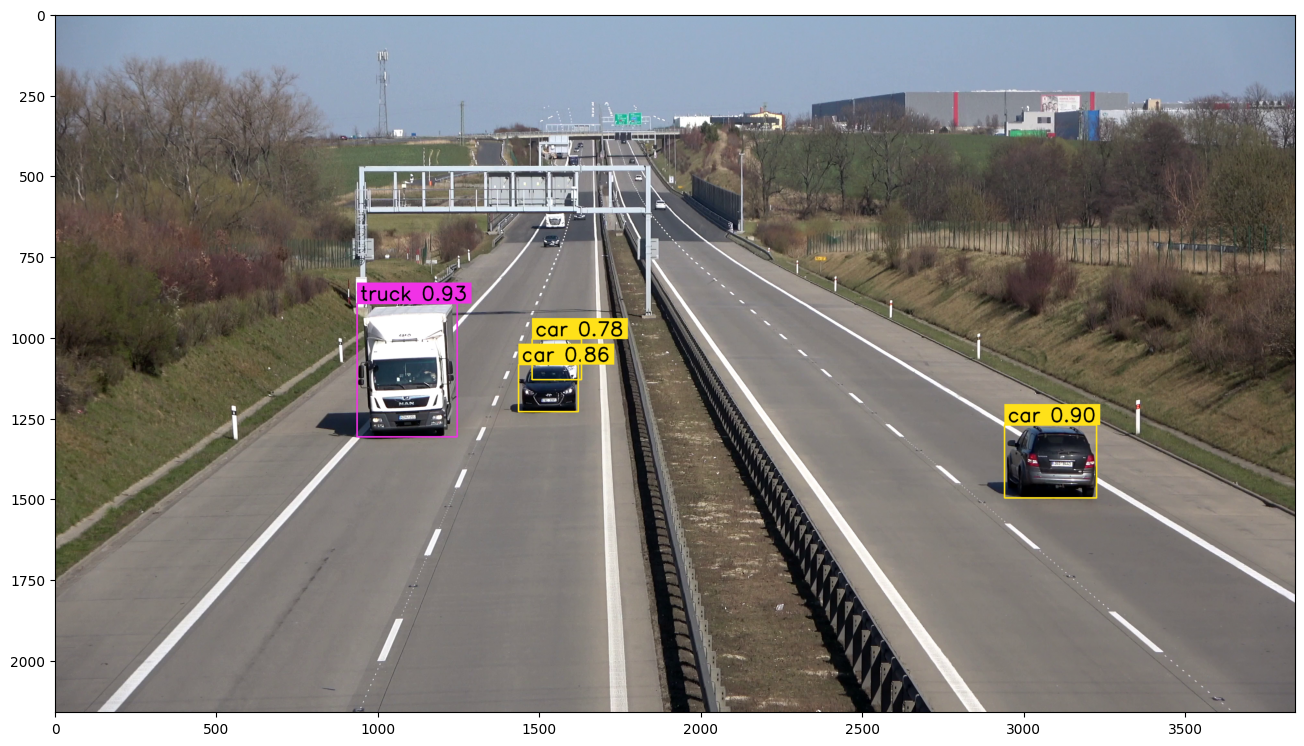
预测标注整个视频#
# settings
LINE_START = Point(50, 1500)
LINE_END = Point(3840-50, 1500)
TARGET_VIDEO_PATH = f"{HOME}/vehicle-counting-result.mp4"
VideoInfo.from_video_path(SOURCE_VIDEO_PATH)
VideoInfo(width=3840, height=2160, fps=25, total_frames=538)
import numpy as np
# 添加 np.float 属性
if not hasattr(np, 'float'):
np.float = float
# 然后运行你的代码
from tqdm.notebook import tqdm
# create BYTETracker instance
byte_tracker = BYTETracker(BYTETrackerArgs())
# create VideoInfo instance
video_info = VideoInfo.from_video_path(SOURCE_VIDEO_PATH)
# create frame generator
generator = get_video_frames_generator(SOURCE_VIDEO_PATH)
# create LineCounter instance
line_counter = LineCounter(start=LINE_START, end=LINE_END)
# create instance of BoxAnnotator and LineCounterAnnotator
box_annotator = BoxAnnotator(color=ColorPalette(), thickness=4, text_thickness=4, text_scale=2)
line_annotator = LineCounterAnnotator(thickness=4, text_thickness=4, text_scale=2)
# open target video file
with VideoSink(TARGET_VIDEO_PATH, video_info) as sink:
# loop over video frames
for frame in tqdm(generator, total=video_info.total_frames):
# model prediction on single frame and conversion to supervision Detections
results = model(frame)
detections = Detections(
xyxy=results[0].boxes.xyxy.cpu().numpy(),
confidence=results[0].boxes.conf.cpu().numpy(),
class_id=results[0].boxes.cls.cpu().numpy().astype(int)
)
# filtering out detections with unwanted classes
mask = np.array([class_id in CLASS_ID for class_id in detections.class_id], dtype=bool)
detections.filter(mask=mask, inplace=True)
# tracking detections
tracks = byte_tracker.update(
output_results=detections2boxes(detections=detections),
img_info=frame.shape,
img_size=frame.shape
)
tracker_id = match_detections_with_tracks(detections=detections, tracks=tracks)
detections.tracker_id = np.array(tracker_id)
# filtering out detections without trackers
mask = np.array([tracker_id is not None for tracker_id in detections.tracker_id], dtype=bool)
detections.filter(mask=mask, inplace=True)
# format custom labels
labels = [
f"#{tracker_id} {CLASS_NAMES_DICT[class_id]} {confidence:0.2f}"
for _, confidence, class_id, tracker_id
in detections
]
# updating line counter
line_counter.update(detections=detections)
# annotate and display frame
frame = box_annotator.annotate(frame=frame, detections=detections, labels=labels)
line_annotator.annotate(frame=frame, line_counter=line_counter)
sink.write_frame(frame)
展示前面保存的视频#
在视频处理完成后添加以下代码#
from IPython.display import Video
展示生成的视频#
Video(TARGET_VIDEO_PATH, embed=True)